Metrics to Select and Measure Twitch Creators for Game Marketing- Part 2
In this second part, we will walk you through some of the more complex metrics we use at Gamesight when finding and measuring influencers for video game marketing campaigns.
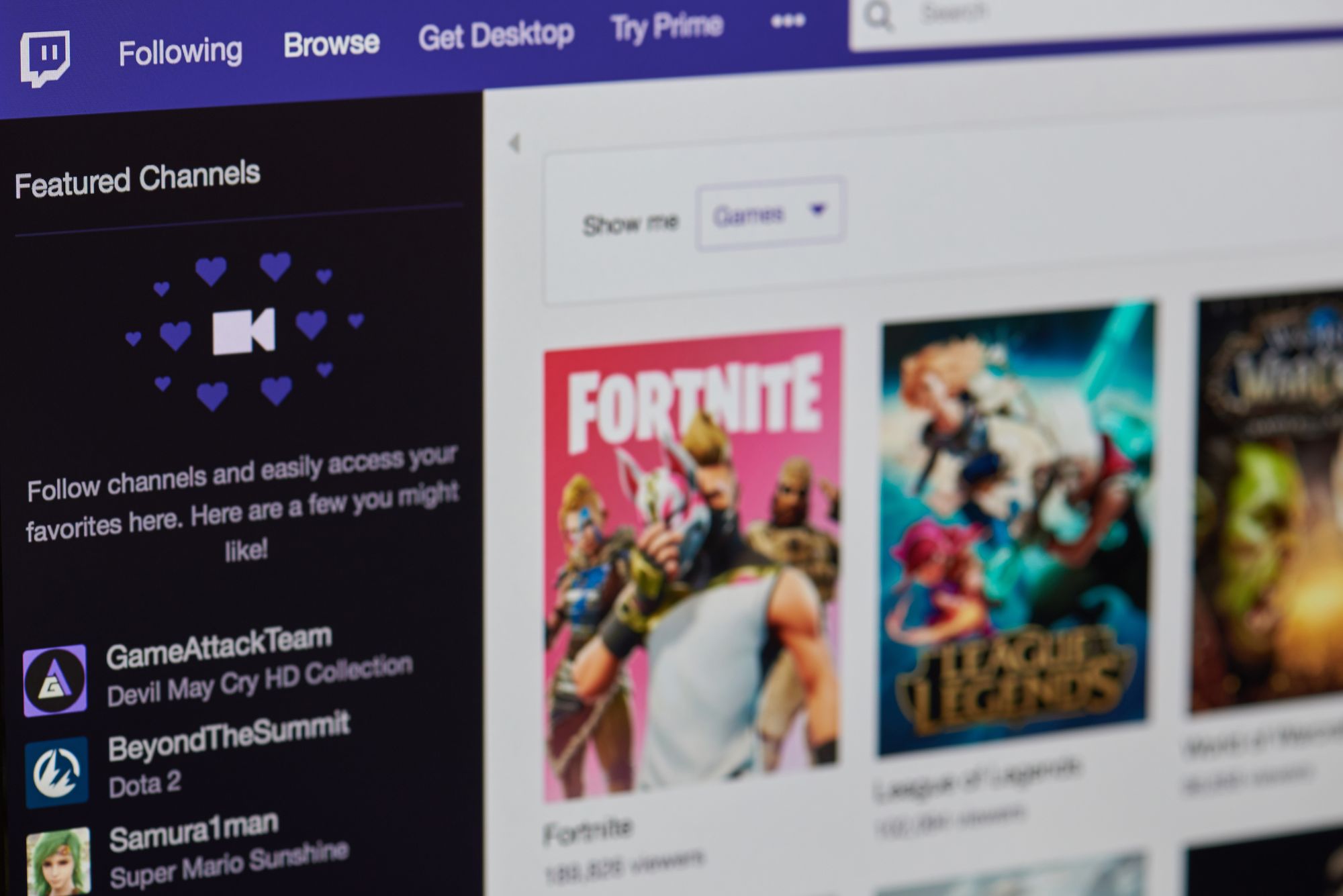
This article is the second part in our series on metrics for Twitch channels. In part 1, we discussed simple Twitch metrics like follower counts, VOD views, channel views, and peak concurrent viewers. In this second part, we will walk you through some of the more complex metrics we use at Gamesight when finding and measuring influencers for video game marketing campaigns.
Average Concurrent Viewership (ACV)
Average concurrent viewership represents the average number of viewers watching throughout a stream. In other words: a live stream’s viewership is repeatedly sampled, and after the stream is over, this viewership is summed up and averaged over the total stream duration.
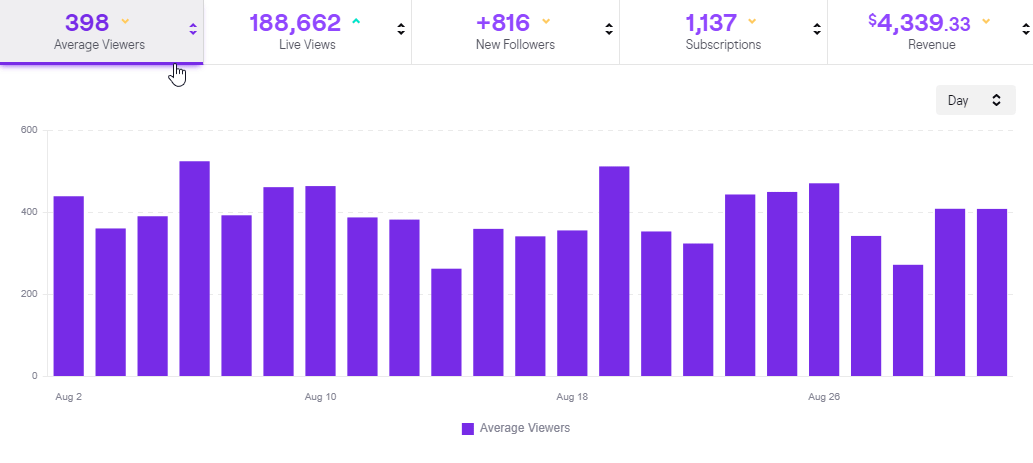
The ACV is accessible to creators through their internal Twitch dashboard and is used to benchmark a creator’s engagement. Twitch itself requires at least three concurrent viewers for channels to join their affiliate program and at least 70-80 for their partner program.
In the following graph, you can see a single 7-hour stream that contained two games: the first game (blue) had high viewership, but at one point the stream switched over to a second game (yellow), and viewers quickly dropped off.
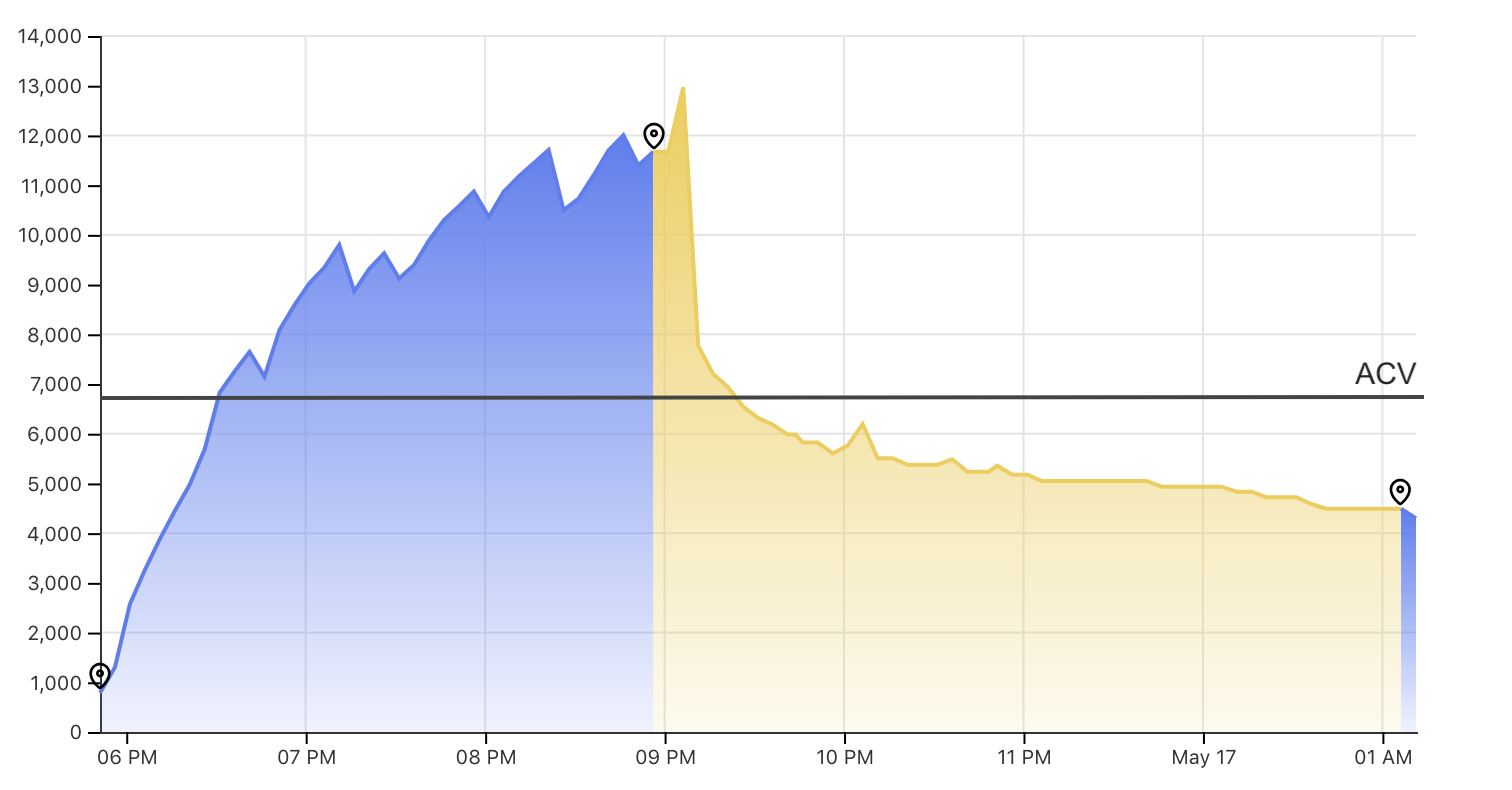
This stream had a peak viewership of over 13,000, but the ACV for the stream is around 6,800.
Benefits
ACV is a measure of engagement, quantifying how much attention a streamer retains when streaming. If people leave after watching for a few minutes, it lowers a channel’s ACV, showing that the streamer is not maintaining viewer engagement.
In the above example, using ACV and peak viewership together gives you a quick assessment of a stream’s “shape.” If you see that a stream has very similar peak viewership and ACV values, you know that the stream’s viewership didn’t fluctuate much.
Another benefit is that ACV is relatively unaffected by short boosts in viewers compared to peak viewership. The metric levels out the effect of viewers who watch for very little time in favor of viewers who continue to watch for longer. In other words, unlike peak viewers that count regardless of watch time, ACV adjusts as viewers come and go.
Drawbacks
ACV can be an underrepresentation of a stream’s total reach since it does not account for changes in who is watching the stream over time. For instance, an hour long stream with 100 ACV could either mean that the stream saw 100 unique viewers, each watching for one hour or 200 unique viewers, each watching for 30 minutes. Because of this, average viewership should be interpreted while taking into account metrics like peak viewership to assess the stream’s engagement and reach.
It would be overly simplistic to discuss the viewership of an entire 24-hour long stream using a single number. ACV becomes less valuable as a standalone metric the longer a stream goes on. Longer streams retain fewer viewers on average as they progress, as few people can stay engaged with content for several consecutive hours on end. This means longer streams almost always have lower ACV than shorter streams.
The chart below visualizes this pattern. It shows the length of a stream against the ACV adjusted for a channel’s follower count, comparing ACV independent of how big a channel is. Streams that are two hours or shorter generally have the highest ACV, and longer streams almost always have lower ACVs.
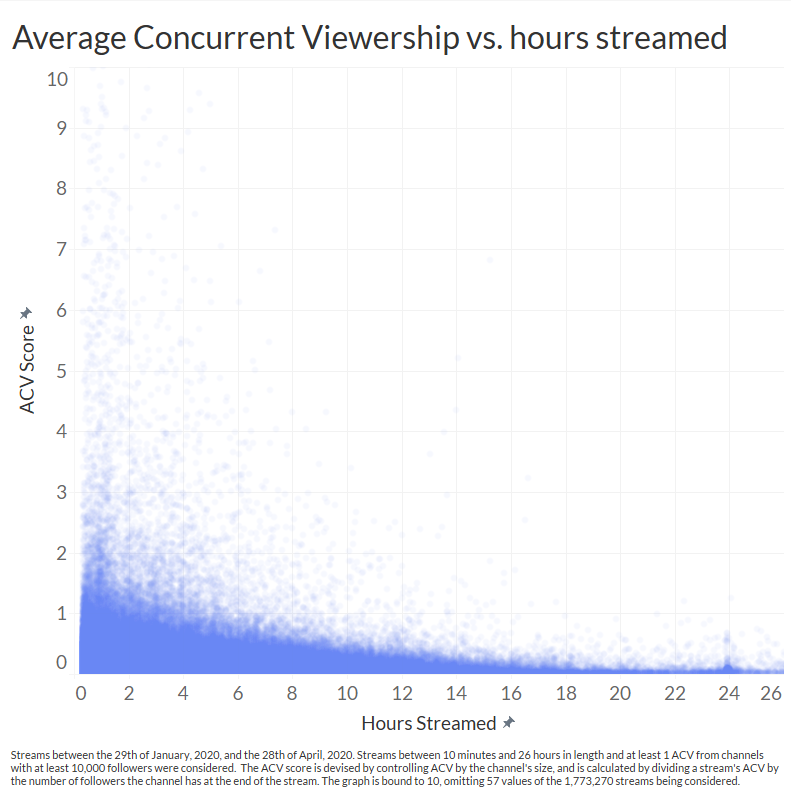
Viewer-hours
Viewer-hours is a measure of how many hours of content were watched during the stream. For example, if a 2-hour long stream had 100 viewers for the whole stream, it would have had 200 viewer-hours. Viewer-hours can be calculated easily by multiplying ACV with the stream’s duration in hours.
This is one of the most valuable pieces of information about live content. When compared to viewership, it actually takes into account how “much” of the content was consumed. For instance, 100 people could have watched a stream, but using viewer-hours can inform on whether they watched the stream for 5 minutes or 5 hours.
Another benefit is that viewer-hours can also be meaningfully added across streams to calculate total content consumption over time. This enables you to compare games, creators, and streams directly even if they produce different amounts of content. For instance, assessing how much of Fortnite’s watchtime was contributed to by different creators is impossible using ACV or viewership alone.
Drawbacks
Viewer-hours alone can’t be used to differentiate between a large number of lightly engaged viewers versus a smaller number of very loyal viewers. A stream with 100 viewer-hours can either be composed of 100 viewers who all watched for a single hour, or one viewer who watched the stream for 100 hours. Like ACV, this can be accounted for by using other metrics like viewership.
This means it is also best used as a metric when focusing on viewer engagement campaigns. Our data shows that the likelihood of a viewer trying out a game falls off greatly after the first few hours of watching content. If you are running a user acquisition campaign, you want to be focused on measuring the number of new viewers you are reaching. Relying too heavily on viewer-hours as a key metric will lead you to run longer streams that have greatly diminishing returns.
Deep dive: example of viewer-hours and ACV in action
Twitch Drops was introduced in 2017 and rewarded viewers who watch streams for some time with in-game rewards. This system saw its first wide-scale use with Warframe’s “Great Eidolon Hunt” - a campaign that offered exclusive in-game rewards like cosmetics, currency, resources, and weapons for watching the game’s partnered streamers.
The campaign ran from October 12th, 2017 to November 2nd, 2017. During the entire course of the campaign, content consumption as measured by viewer-hours increased ~13x, whereas average concurrent viewership increased ~3x. This suggests that engagement across Warframe streams increased significantly due to viewers staying engaged longer, not necessarily that the streams were reaching significantly more new total viewers.
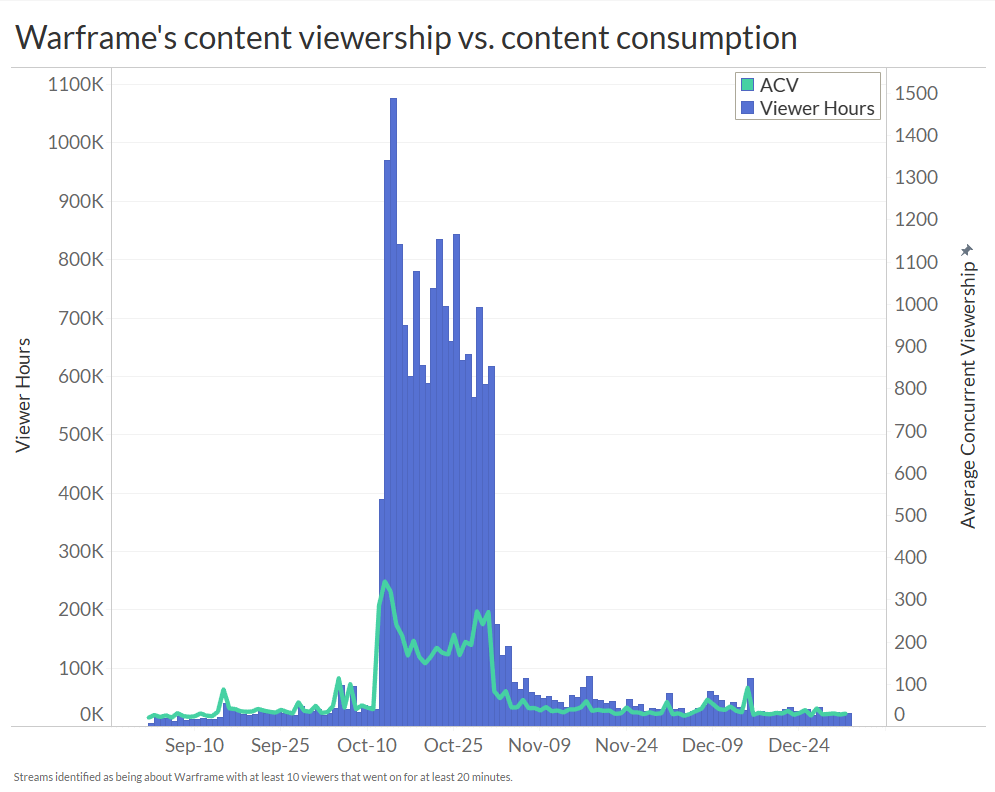
By using ACV and viewer-hours together, you can start to understand the difference between campaigns that bring new audiences to a game compared to those that engage existing viewers. Many of the advanced metrics that we discuss in the next section are designed to help dive deeper into these types of questions.
Advanced Metrics
The above metrics provide an excellent foundation for getting started with influencer measurement and marketing. As you get deeper into using data to measure your campaigns, the most common trap people fall into is chasing large numbers rather than impactful ones.
Setting goals and clearly defining your objectives upfront is the key to success. Decide whether you want to reach new users or engage with your existing community first because it has a considerable impact on the strategies and metrics that you will use.
As a part of executing, measuring, and persistently analyzing thousands of influencer campaigns over several years, we have developed several metrics that we use to assess channels. This section introduces a few questions and more in-depth metrics to consider while you are planning your campaigns.
Growth
One of the most valuable things you can do with any of the metrics we have discussed in this series is observing how they change over time. For instance, if a channel’s follower count or ACV is suddenly doubling weekly, they could be a channel worth looking into. Conversely, identifying channels that are flatlining can be great for finding channels that have hit growth hurdles and are actively seeking ways to diversify their content. In either case, looking at growth can help you identify hidden gems that other people miss.
Streamer Freshness and Audience Health
If a channel has previously streamed your game, either organically or through partnerships, the majority of their audience has likely already decided whether they want to play it. On the other hand, if a streamer’s viewership is stagnating or decreasing, this can be an indicator that the audience is burning out on their content.
While these channels may be great for community engagement, working with either set is likely a bad idea if your goal is user acquisition. Identifying the freshness of a streamer’s audience by analyzing their audience and engagement changes over time is important when determining the right time to be working with an influencer. By comparing historical data, it’s possible to estimate the creator’s burn-in or audience stagnation, and the effect of repeated activations from working with the same channel again. This foundational analysis that we do at Gamesight is designed to ensure that every campaign works with the right channels for its goals.
Audience Loyalty
Some streamers have audiences that are very sensitive to new games or sponsorships. For instance, some creators specializing in certain games have die-hard followings that only want to see them play the game they specialize in. On the other hand, variety streamers often have audiences that tune in specifically to watch the streamer try new games and see new content.
Partnering with streamers whose audience isn’t a good fit for your game is bad for everyone. The streamer loses trust with their audience and you make the wrong impression, all while wasting valuable marketing budget. To that end, considering how a channel’s viewership changes between different games and content types is vital to ensure you’re getting the most out of the relationship.
Looking at viewer dropoff is one of the most effective ways of projecting whether a channel is a good fit. You can calculate dropoff as a percentage of viewership lost when the channel streams a game similar to yours compared to their primary game(s). Keep this number in mind and set performance expectations upfront.
Game similarity
The games an influencer plays reflect the interests of their pertaining audiences. For instance, someone who mostly plays Need for Speed or Forza Horizon would likely be a poor fit for a campaign promoting a Call of Duty. It is essential to identify which genres fit your game, find matching games based on this, and try working with influencers who play these games. Doing so will tailor your promotion to audiences who are already interested in your game genre, and who may want to buy it after watching it streamed.
From our analysis, each genre may have thousands of games spanning back several decades that are still actively being played on Twitch. It is time-consuming to catalog these games and the channels playing them, but doing so can help you find smaller channels with very loyal audiences dedicated to games like yours. Micro-influencers tend to have much higher engagement relative to the size of their audience and can provide tremendous value to the campaigns they are a part of.
Closing Thoughts
There is no magical, all-powerful single metric that perfectly captures which creator is the best for your game. This undertaking requires using all of the metrics to maximize their strengths and involves answering different questions tailored to finding the right influencer for you. The answer will always change based on your game, budget, start time, and even platform (YouTube, Mixer, Twitch, Instagram, etc.). We hope this article will, at the very least, help you consider some of the tools you can and should be using when deciding to work with influencers.
At Gamesight, we are continually testing and evolving this process, often adding new ways to measure influencers to our metrics repertoire. We use each of these metrics among several other, even more, data-driven ones to identify and measure influencers for our partners. If you would like to speak with us about this article, or are a developer or publisher and want to work with us for your advertising needs, we would love to hear from you! Please reach us at support@gamesight.io.